Research & development
Rooted in our scientific heritage at the EESD group at EPFL, we are dedicated to advancing state-of-the-art technologies in image analysis, 3D computer vision, AI, and drone automation for the digital inspection of structures. Through continuous improvement of our processes and algorithms, we ensure robust and precise results. Our commitment to research and development, in collaboration with leading institutions like EPFL, keeps us at the forefront of innovation, driving digital transformation in the AEC industry.
On this page, we share recent R&D projects in which SwissInspect has been actively involved, showcasing our contributions to advancing digital inspection technologies.
Projects
Intelligent Bridge Inspection
Funded by innosuisse
The "Intelligent Bridge Inspection" project received support from Switzerland's most prestigious innovation agency, Innosuisse. Conducted in collaboration with the EESD group at EPFL, the project included a structural testing campaign. Alongside our scientific partner, the Federal Office of Roads of Switzerland and Kanton Zürich contributed as expert industry partners.
This project focused on advancing three core pillars of image-based bridge inspection solutions:
- Automation and Optimization of Data Collection: Leveraging industry-grade drones for efficient and repeatable data collection.
- Computer Vision and AI Analysis: Enabling automated creation of damage-augmented digital twins for detailed structural assessments.
- Scalable Web Platform Development: Building a robust platform for managing and scientifically visualizing multi-modal data tailored for structural engineers.
A key emphasis of this project was on open data format practices, ensuring integration and communication of image-based inspection results with BIM software solutions and other existing database management systems within organizations.
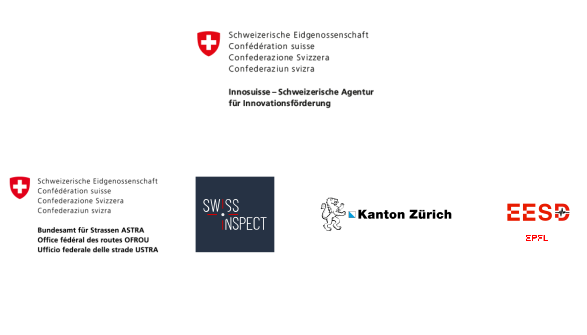
Automated Augmentation of Building LOD models
Consortium Guichet carto 3D | Funded by SyNNergy program-innovaud
While building inspections are not regulated to the same extent as infrastructure inspections (e.g., bridges), they remain crucial for government authorities and engineers. Accurate building inspections, whether at an individual building scale or at a neighborhood/city scale, are essential for tasks like urban planning, risk assessment (e.g., flood or avalanche risks), and city development strategies. To make this information interpretable, storable, and easily communicable, simplified semantic city building models are becoming increasingly important. These models provide an abstract representation of buildings while retaining the key details necessary for analysis and decision-making.
However, one significant challenge lies in automating the creation of augmented Level of Detail (LOD) models from image-derived 3D meshes, often produced using photogrammetry methods. In the collaborative project "Guichet Carto 3D" funded by the SyNNergy program at Innovaud, several technological solutions were developed—ranging from effective data visualization to the creation of semantic information from 3D photorealistic meshes.
SwissInspect as one of the partners of this project was responsible for the development of semi-automated and automated methods for augmenting LOD building models, including widely-used datasets like SwissBUILDINGS3D.
The video shows the creation of a textured 3D model from aerial images taken by a drone. We developed an approach for augmenting the existing LOD models (including the openings) using the registered camera positions from the structure-from-motion pipeline. The image below shows several views of the augmented LOD building models.
Scientific publications 📝
Crack segmentation: Reduce annotation effort smartly
AI models thrive on data—but not just any data, annotated data. Training a robust model for structural defect detection, especially for crack segmentation, typically demands extensive pixel-level annotations. Now, imagine manually labeling every crack pixel across thousands of images—it is an overwhelming task.
But do we really need to annotate every pixel? Is there a smarter way to reduce the annotation effort without sacrificing performance?
The answer is yes. In our article, "Partial Annotations in Active Learning for Semantic Segmentation," published in the Automation in Construction journal, we demonstrated that it is possible to achieve the same level of performance in crack segmentation while using only a fraction of the training data. This is not only for cracks, but also other damage types like corrosion, or to be more precise, for any other segmentation task!
This approach significantly reduces annotation efforts without compromising the accuracy of the AI model—offering a smarter, more efficient pathway for developing AI-powered inspection tools.
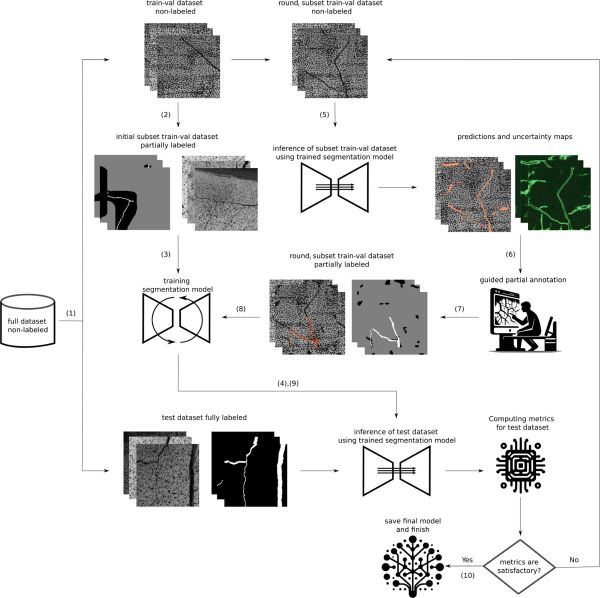
Uncertainty quantification in crack segmentation and quantification
Imagine you have a database of crack images paired with their pixel-level ground-truth masks. You train your AI model—let’s say an encoder-decoder architecture—following best practices like cross-validation. You achieve an impressive segmentation score and feel confident in your results. But here’s the question: Is this enough to meet the standards of structural engineering best practices?
The answer is no. One critical step is often overlooked: uncertainty quantification. In our study, we tackled this challenge by exploring uncertainties in the detection and quantification of cracks. We designed an experimental campaign testing concrete beams, evaluating two key types of uncertainty: i) model uncertainty; and ii) aleatory uncertainty: factors such as camera angle, noise, blurriness, and other environmental variations.
Beyond that, we proposed a new evaluation metric specifically tailored for crack segmentation, one that goes beyond traditional computer vision metrics like the F1 score.
Ready to collaborate
We are passionate about advancing AI, 3D computer vision, and structural inspection technologies, and welcome collaborations with academia and industry partners for impactful projects.